July 1, 2022
•
...
A long, not so long time ago, when I was a happy-go-lucky college freshman taking my first finance class getting preached the Efficient Market Hypothesis, I remember wondering: “If markets are efficient and perfectly represent all available information, what’s the point of day-trading? How can you beat a perfect price?”
After a bit of experience, it of course starts seeming like a silly question. Every trader knows the EMH is not saying an asset is perfectly priced to where there is no reason for prices to move outside of new information. Rather, assets are simply priced where the flow was last balanced- where the last buyer found a seller or where the last seller found a buyer. (Implicitly, in a zero-sum game like options, this also means the point where there is an equal number of buyers as sellers). Every participant doesn’t necessarily agree on the current price- it just means they haven’t executed a trade the other way yet, whether that be due to risk limits, lack of/inaccessible capital, indecisiveness, not staring at the markets 24/7, needing their manager’s approval, etc. As a trader, one framework for finding +EV trades is identifying when the market price is “offsides” and will be pushed back when these various inefficiencies get resolved with time.
Even if we lived in a world where every participant was happy and executed all the trades they wanted to, this “efficiency” wouldn’t be equivalent to a “perfect price”. In order for that to be true, each participant would need to be equally adept, i.e. each participant picks the winning trade 50% of the time (assuming each trade makes the same $/trade). So now, much like table selection in poker, an important piece in the game of trading is finding the markets where participants win at the lowest possible rates- aka where one feels like they have some sort of “edge” over the population.
Where can we find markets that are both offsides often and have weak participants?
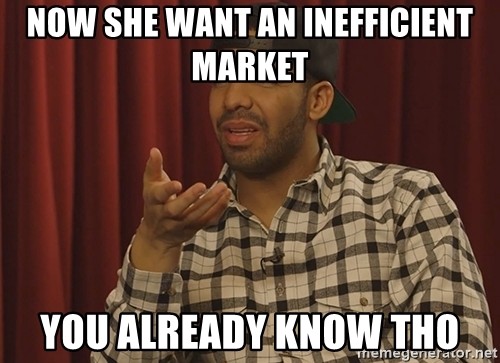
The purpose of this article will be examining a few ways in which crypto markets are inefficient and why some participants win at low rates.

Capital, Regulation, and Market Fragmentation
Historically, crypto trading is primarily a game of capital. This stems from market fragmentation + regulations. The most obvious example is the early arb opportunities across spot markets in popular exchanges. If a Coinbase BTC buyer comes in and continually bids up 50m of spot BTC on just Coinbase, BTC prices on Coinbase vs other exchanges will start to diverge. In a world with infinite capital, the divergence would be tiny- market participants would be happy continuously selling Coinbase $.01 over where they can buy elsewhere. But in the real world, the divergence would increase heavily as participants slowly run out of BTC to sell on Coinbase and must transfer over cash from Coinbase-> elsewhere and BTC from elsewhere->Coinbase.
The spot arb is almost definitely a pure arb that will get closed with time(outside of black swan events such as an exchange going under and not giving back user funds). Similar arbs can happen in vol-space across options markets, as participants must margin each exchange individually and are thus capital-constrained on the absolute position they can hold on each exchange. Why might options arbs take longer to close? Unfortunately, you cant simply send an options position from one exchange to another as you can with physical coins.
I consider this capital arb to go hand in hand with regulation arb- a primary reason driving the lack of capital is traditional firms being slow to deploy capital, first needing to navigate the regulations involved. Its easier for a crypto native firm without a large entrenched tradfi business to throw caution into the wind and deploy capital as they have less to lose.
As always, there’s a relevant MGNR tweet that summarizes the whole thing, in this case referencing the basis trade version of capital arb:
The lack of capital pushing prices in lines can lead to some well-documented inefficiencies. The most discussed is the concept of “forced sellers and buyers”, mostly referencing when liquidations cause market volatility, which in turns causes more liquidations- a phenomenon known as cascading liquidations. With only a certain amount of capital providing liquidity at a given moment, common wisdom (likely correctly) dictates that trading vs. forced flow is +EV as capital will eventually come back in to absorb the flow and push prices back to normalcy. In the options world, we should then look for forced buyers and sellers as prime meat to trade against. Options obviously can have liquidations as well- but besides that, in the current paradigm, we have weekly forced sellers of options in the forms of Defi Option Vaults(DOVs). These DOVs have a certain amount of capital to unload onto the markets every week in about a 12 hour timespan. Here is a great article by the Friktion team discussing the impact of this capital on the markets. Lucky for us, if we want to get involved in trading vs. the forced DOV flow, Paradigm is doing great work integrating with the vaults- see Ribbon,Thetanuts, and Friktion.
Another area that I would classify as “forced sellers” that we may see come out of the woodworks are yield-starved option sellers. As the bear market wanes on and participants(such as miners struggling to make ends meet at low BTC prices) search for ways to stay afloat, they may give away their upside convexity exceedingly cheap to lock in some guaranteed cash. In a miner’s case for example, they could continually roll the following trade: sell X monthly call options for a 1% yield, where X is the expected number of bitcoins mined per month. I imagine we will find some fire-sale bargains from an implied volatility perspective in the 5–15 delta call buckets over the next 2–6 months.

Pricing issues in a nascent market
Let’s take a look at interesting pricing concepts in crypto options.
Imagine you were tasked with pricing an option on BTC in 2010, when there was no liquid options market on any crypto. How would you do it? The simplest way would obviously be to borrow from tradfi: plug in the asset to a Black Scholes (BS) model(or your favorite variation) and perhaps borrow the assumptions we use in equities markets. Now here are the problems you may face:
1. Lack of historical data to price Implied Volatility
If you only have a couple years and one regime to calculate realized vol, how can you price implied vols? How do you price skew when narratives are constantly changing, i.e is btc a commodity with a call skew or an equity with a put skew? How do you price how IV/RV will react to new events we don’t have data for (world wars, pandemics, fed rate hikes, crypto hacks, halvenings, miner bans)? As a trader, forming a strong opinion on any of these will give you an edge over a new market struggling to price the unknown.
2. Problems with BS itself
Does BS properly price implied volatility for a reflexive, sometimes liquidation driven asset? The answer is likely no. Prior to the meltdown over the past week, BTC had seen local lows of 26k. If one could form a hypothesis using their favorite dataset/astronomy sign/CT guru/TA fibonacci sequence that we would see cascading liquidations resulting in a 10% downtick if prices hit 24k, this can have interesting effects on the vol smile. All of a sudden, instead of a traditional smooth vol curve that one may look at in delta(or probability) space, it can start having vol bumps in strike space. The 24k liquidation point hypothesizers may be willing to pay a premium for specifically the 24k strike because reaching the strike will trigger high realized volatility. Maybe they take advantage by buying the 20k/24k/28k put fly- buying 2 of the “underpriced” 24k strike on a smooth curve and selling the 1 of each the 20k and 28k strikes which are more fairly priced. Worry not if that’s confusing, because have included my excellent Microsoft Paint drawing of this effect below.
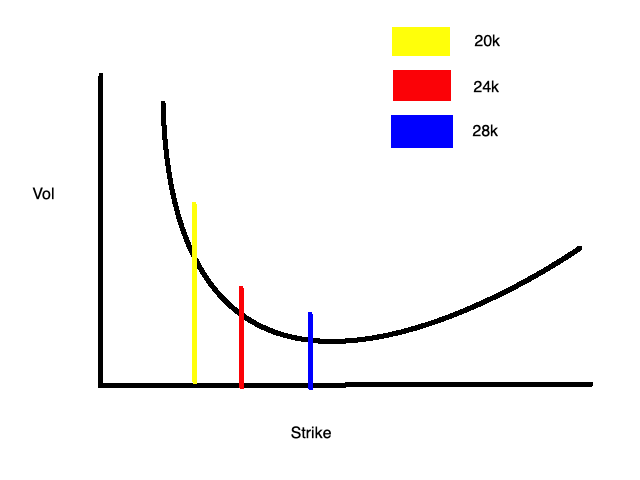
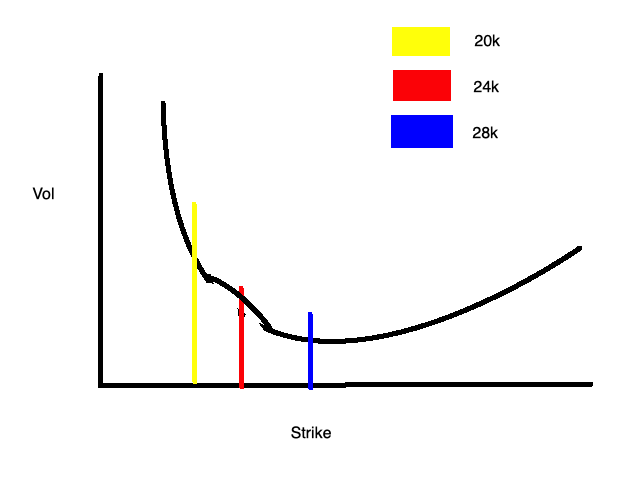
Reflexivity(and/or auto-correlation) can cause similar problems to traditional options pricing. For example, if in a vacuum you believe there is a 1% chance BTC hits 150k and a 3% chance it hits 100k by EOY, but also that the probability that (BTC hits 150k given it already reached 100k) is disproportionately high, this can cause another degree of difficulty in pricing.
Read more on reflexivity from rather large-brained traders Avi Felman and Adi Dommaraju here. Or if you prefer hearing Mr. LightCrypto say the word reflexive instead, here’s an omniscient tweet during the early stages of the 2020 bull run from him:
Case study: Jan 2021 36k calls
A legendary trade in late 2020 was a buyer of size BTC January 2021 36k calls. The background on the trade can be found here, but the important part is someone lifted 16k of the far, far out-the-money calls when bitcoin was trading 13k and then exited the trade a few months later for a massive profit with bitcoin well north of 30k. The reason I bring this trade up is that one could, with the luxury of hindsight, hypothesize that the seller of these options heavily mispriced the options with respect to how much power reflexivity/liquidations could have on spot price. At the time, 36k was the highest strike listed on Deribit and most watching would laugh off buyers of the strike as hopelessly optimistic.
With the ETH merge fast approaching, the options market has already begun to see bets on how its being priced.
Unlike most programmed tradfi events (CPI, nonfarms, fed hikes), crypto events such as the halvening and the merge don’t simply print a number and play out instantaneously. While the merge does have some binary-ish outcome component to it (fail/success/delay), most of the event will play out over the following weeks to months as market participants react to the changes. It will be left as an exercise to the reader on whether they believe there are Jan 2021 36k call-like opportunities betting on upside convexity for the merge.
Finale
Most of the examples I discussed are inefficiencies of the past. But as crypto continues to evolve and new tokens, events, narratives, exchanges, and products(squeeth anyone?) are created, there are going to be opportunities driven by the same basic foundations: limited capital and regulations limiting certain participants, risk limits + volatility limiting position sizing, fundamental misunderstandings of the pricing of nascent products/asset classes, and of course, a subset of participants just looking to scratch a gambling itch and pushing prices offsides.
Heading 1
Heading 2
Heading 3
Heading 4
Heading 5
Heading 6
Lorem ipsum dolor sit amet, consectetur adipiscing elit, sed do eiusmod tempor incididunt ut labore et dolore magna aliqua. Ut enim ad minim veniam, quis nostrud exercitation ullamco laboris nisi ut aliquip ex ea commodo consequat. Duis aute irure dolor in reprehenderit in voluptate velit esse cillum dolore eu fugiat nulla pariatur.
Block quote
Ordered list
- Item 1
- Item 2
- Item 3
Unordered list
- Item A
- Item B
- Item C
Bold text
Emphasis
Superscript
Subscript